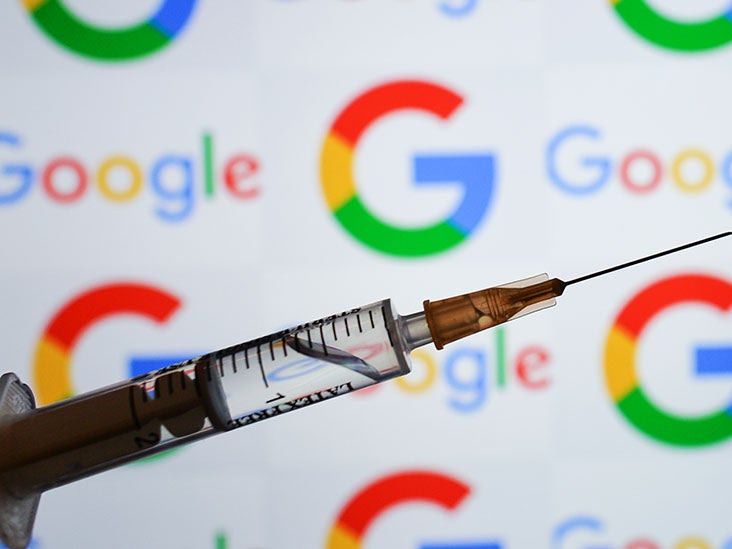
- New research finds that online searches can accurately predict regional increases and decreases in COVID-19 cases.
- Certain types of searches reveal the activities in which people plan to engage.
- The search volume for outside-the-home vs. stay-at-home activities forecasts the number of COVID-19 diagnoses 10–14 days later.
While some of the behaviors that lead to SARS-CoV-2 infections are clear, new waves of COVID-19 cases do not always follow predicted patterns.
Now, however, a study from researchers at New York University’s Courant Institute of Mathematical Sciences describes a possible means of spotting infection surges before they happen through the analysis of online searches.
The researchers discovered a correlation between a surge in searches relating to activities outside the home — activities that could put people at risk of SARS-CoV-2 infection — and a rise in COVID-19 cases 10–14 days afterward. Infections fell when there was an increase in searches relating to stay-at-home activities.
Study author Anasse Bari, a clinical assistant professor at the Courant Institute, notes that experts have already successfully used data mining “in finance to generate data-driven investments, such as studying satellite images of cars in parking lots to predict businesses’ earnings.”
“Our research shows the same techniques could be applied to combatting a pandemic by spotting, ahead of time, where outbreaks are likely to occur,” says senior author Megan Coffee of the Division of Infectious Disease & Immunology at the New York University (NYU) Grossman School of Medicine.
Identifying with greater precision those behaviors that produce infection spikes can help epidemiologists and policymakers more effectively shape public policies regarding closures, lockdowns, and so on.
The system that the study paper describes avoids privacy issues by involving only large clusters of anonymized data.
The study appears in Social Network Analysis and Mining.
The researchers’ first step was to develop categories based on search phrases or keywords that they could then track.
The two key categories that they tracked were called the mobility index and the isolation index.
The team assigned certain searches to the mobility index track, including “theaters near me,” “flight tickets,” and other inquiries about activities that involve leaving the home and being in physical proximity with others.
As Bari puts it, “When someone searches the closing time of a local bar or looks up directions to a local gym, they give some insight into what future risks they may have.”
For the isolation index track, the researchers collected search queries — such as “at-home yoga” or “food delivery” — that indicated an intention to remain home and isolated.
The researchers based their categorization of keywords on the Democracy Fund + UCLA Nationscape survey — a study in which respondents listed the things that they would be doing if “restrictions were lifted on the advice of public health officials regarding activities.”
The survey found that the top three activities that people missed were “going to a stadium/concert,” “going to the movies,” and “attending a sports event.”
According to Bari, “This is a first step toward building a tool that can help predict COVID-19 case surges by capturing higher risk activities and intended mobility, which searches for gyms and in-person dining can illuminate.”
The researchers collected search data for March through June in 2020 from all 50 states in the United States. They used Google Trends to track trends in the data, allowing them to develop the mobility and isolation indexes.
The researchers also created a “Net Movement Index” to indicate the relationship between the two indexes. A higher Net Movement indicated a shift toward mobility search queries and away from isolation searches.
The authors write, “We theoretically expect that a sudden decline in net movement (i.e., more people staying home) would correspond to a reduction in COVID-19 spread, with a lag equivalent to the incubation period of COVID-19.”
In 42 of the 50 states, each rise in Net Movement accurately predicted an increase in COVID-19 infections 10–14 days later.
The authors of the study explored the relationship between the mobility index and infection rates following the removal of stay-at-home orders in five states: Arizona, California, Florida, New York, and Texas.
Following the implementation of each lockdown, the mobility index had significantly decreased, mirrored closely by a reduction in infections. However, the easing of stay-at-home orders in Arizona, California, Florida, and Texas preceded a sharp increase in the mobility-type searches, followed shortly by a spike in the number of reported infections in June 2020.
Another author of the study, NYU undergraduate Aashish Khubchandani, concludes:
“From this work, we hope to build a knowledge base on human behavior change from alternative data during the life cycle of the pandemic in order to allow machine learning to predict behavior in future epidemics.”
For live updates on the latest developments regarding the novel coronavirus and COVID-19, click here.
"how" - Google News
February 20, 2021 at 03:26PM
https://ift.tt/2OZu429
How Google search data can predict COVID-19 outbreaks - Medical News Today
"how" - Google News
https://ift.tt/2MfXd3I
Bagikan Berita Ini
0 Response to "How Google search data can predict COVID-19 outbreaks - Medical News Today"
Post a Comment